President and Cofounder, Partner
Artificial intelligence in marketing: Get ready for the trough of disillusionment
President and Cofounder, Partner
If for the past year or so there’s been one subject on everyone’s lips at marketing and business events, it’s been artificial intelligence. What awaits this techy buzzword?
The hype cycle
Like many other inventions before it, artificial intelligence is a technology that should be subject to the same cycle of adoption, according to Gartner’s now-celebrated hype cycle.
In my opinion, we’re currently scaling the upward slope of inflated expectations. All the symptoms of hype are present, including the following:
- It’s a subject presented at most business and marketing conferences.
- Its key terms— artificial intelligence, deep learning, machine learning, algorithms, automation, and many others—are used over and over again to the point of cliché.
- Dozens of companies are announcing huge investments and opening R&D facilities focused on AI. In Montreal alone, Facebook, Microsoft, Google, Thales SA, and Samsung have already announced the openings of such exploratory centres.
- Companies are fighting to grab up specialized resources in the domain.
- Many statisticians, mathematicians, and data analysts are reshaping their professional profiles as data scientists in order to ride the wave.
- Companies are investing and hiring, but don’t necessarily have clear or well-established business plans or use cases.
Inevitably, this overblown enthusiasm will lead to disappointment. We’ve already seen this type of hype cycle play out in several areas, notably regarding the web over 20 years ago. The same thing happened with virtual reality a few years ago. (Actually I think we’re still experiencing the trough of disillusionment with regard to that particular technology.)
Disillusionment and the innovation lifecycle
The disillusionment phase obviously brings its share of negative consequences. Projects, programs, and investments are suspended, jobs are lost, and dreams are broken. One could also take the view that these readjustments of the market represent an appropriate return to an equilibrium state where expectations and delivery are more optimally aligned.
In my view, this disillusionment is best expressed by the idea of a group of business managers and researchers who are trying to work together. The managers are looking for a breakthrough concept that will lead to guaranteed profits within a predetermined length of time—generally in the short term. The researchers, however, feel a lot more comfortable with longer term work cycles and indeterminate results, and naturally do not share the same gauge of success. In short, the researchers formulate their hypotheses and see value just as much when those hypotheses are confirmed as when they are negated, as opposed to the managers, who, for a variety of reasons, don’t have a lot of interest in scientifically proving that a particular idea is faulty.
Disappointment will come particularly when these two different realities collide, but also from the process that allows them to get a taste of the advantages of AI. Conferences and discussions surrounding AI focus generally on analysis and data activation, or in other words on insights. But there are two essential phases you need to deploy before you can get there: data capture and engineering. According to three experts invited to a panel discussion on the subject, these two phases represent between 80 and 90 percent of the work in AI.
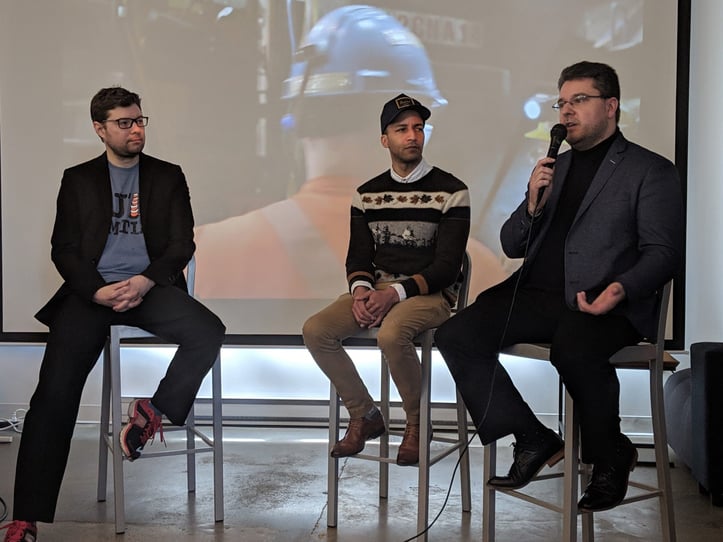
What we see a lot in digital is that data are basically accessible, but most companies capture data in a very approximate way. The situation is even worse when it comes to engineering, which involves organizing, structuring, cleaning up, and preparing data so that the work everyone wants to accomplish can actually begin. Artificial intelligence will only take off if those managing its emergence accept and trust the process.
People developing AI business use cases need to consider this work as part of their costs before vaunting the benefits of AI if they want to grade the downward slope towards the trough of disillusionment.
How to get to the other side of the desert
Consider this picture: There’s a desert you want to cross. Only a few will be successful. Do you trust the people lugging hundreds of litres of water along with them, or those who find a way to create water as they travel? The first group won’t make it to the other side, since they’ll be weighed down by their heavy baggage. These are the companies that hired an AI team too quickly without having a sensible, adapted business plan.
Focus on organizing your data
Concentrate a large part of your work on the capture and organization of data to make them exploitable and reliable. Well-structured data are the oxygen for every AI initiative. Take advantage of the upward slope of the hype cycle, when budgets are larger, to accomplish tasks that will quickly become unpopular when things are sliding down the slope towards the valley of disillusionment.
Read: 7 data marketing principles inspired by Konmari
Focus on the problem
Taking time to properly define the problem will save you time in the end. While it might seem like a good idea to apply emerging technologies or models (chatbots! QR codes! multitouch attribution! influencers!) that may appear to resolve the issue, it’s crucial to ensure that stakeholders have a deep understanding of the problem and have a broad perspective on possible solutions. This is where creativity comes into play, but it cannot thrive without this basic foundation. Who knows, you might even come to the conclusion that you don’t need AI to solve your problem or accelerate your growth!
Focus on developing a culture of exploration and learning from your mistakes
Give your new multidisciplinary teams the freedom to make mistakes since they’re exploring uncertain territory, collaborating together for the first time, and may not always know where their research will take them. For example, don’t take for granted that a mathematician is comfortable with basic marketing principles. The important thing is to establish a culture in which people learn from their mistakes and that encourages the various teams to explore the realities of other involved teams so they can reach a level of mutual understanding that will lead to exponential benefits over time.
Focus first on small projects
Finally, automate the little things and start with simple algorithms that create small advantages. This approach allows you to begin the collaboration process between your researchers (mathematicians, data scientists, etc.) and businesspeople. This step is more than just an end in itself—it’s a way to establish the groundwork for these groups’ crucial collaboration and manage the expectations of those involved. It will also have the effect of decentralizing risk over many small ideas instead of concentrating everything on one big idea whose outcome is too far in the future and too costly. In short, if your team can’t build a cottage in two weeks, there’s a good chance it will never be able to build a castle in twenty years.
Artificial intelligence & Adviso
Our authority isn’t built on basic AI research. Rather it’s our expertise and experience with dozens of different industries and business models that enable us to apply that learning to artificial intelligence in a marketing context. We aim to participate in our clients’ efforts in a concrete way by supporting them during their preliminary work with data, but also through helping them deploy AI use cases that are low risk yet have promising expected returns.
To accomplish this, our data science and technology team applies AI approaches to marketing through dozens of algorithmic models that have demonstrated applications to business issues. For example:
- Identifying the NBA or “next best action”; for example, the next product to suggest to a customer or even the next message to send them in order to achieve the most impact, etc.
- Create a model of custom lookalike audiences that enables you to maximize your new customer acquisition efforts by using a company’s internal data and combining them with online audience data in order to identify the most interesting consumer segments.
- Determine the variables that have the highest impact for our clients in terms of their search engine positioning strategy and consequently redirect our efforts.
When used together, these simple, effective models can also become the basis for a more complex approach that will lead to major differentiation for our clients. But they also have great intrinsic value.
Our digital transformation team supports a range of companies in establishing the aforementioned steps, in creating and organizing the internal teams affected by the advent of artificial intelligence, as well as the marketing and creation of new business models now made possible by AI.