Strategic Development and Marketing Team Lead
5 things marketers don’t know about machine learning, but should!
Strategic Development and Marketing Team Lead
Machine learning. I’m sure I’m not the only one who’s starting to hear this term almost every day… In the news, in your professional life, in heated weekend debates, and from your geekiest, most visionary friends… There’s no doubt, it’s definitely a hot topic these days.
This Google Trends report says it all, showing a growing interest in the subject that far surpasses even the collective excitement generated by programmatic advertising in recent years.
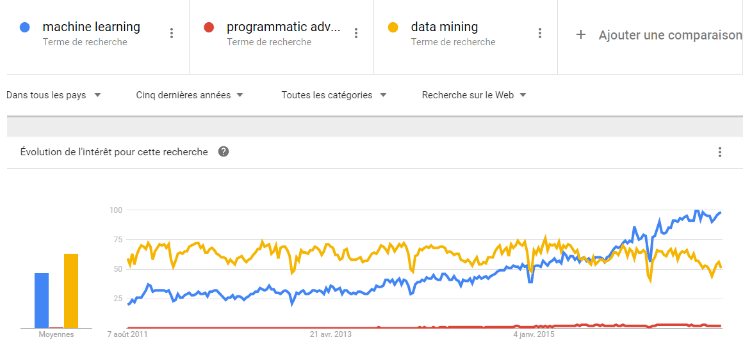
To satiate your curiosity and make sure you no longer need to avoid eye contact when the topic comes up in meetings, we’re going to demystify machine learning (often shortened to ML) in five points. We’ll also explain its impact on digital marketing and media campaigns, and give some concrete examples of how it can be applied. At Adviso, ML has been at the heart of our daily conversations and our digital media toolkit for several months now. We’re going to tell you about the advancements of our Media Innovation and Data Science team (our in-house R&D team) and how their findings could shape the future of your business. And we won’t stop there. This article is the first in a series of publications that will allow you to understand the place of ML in digital marketing, now and in the days ahead.
1 - Let’s start at the beginning: What is machine learning?
Machine learning is a fairly large field of expertise that is currently experiencing a boom. It’s a branch of artificial intelligence.
Although definitions vary from one area of application to the next, machine learning can be explained as a field of study that allows computers to learn and improve automatically based on new data collected in order to make predictions or inferences. Machines are no longer limited to executing commands contained in algorithms, but can learn automatically.
ML can, in some cases, be as narrow as a basic statistical analysis. Essentially, the field of machine learning covers everything from predictive analysis in its simplest form, to reinforcement learning in its most advanced form.
Applied to marketing, predictive analysis uses statistics to predict a given consumer’s behaviour or actions. Predictive analysis allows us to understand what might happen to a particular database in the future, relative to what happened in the past. For example, based on the socio-demographic and behavioural data for a particular pool of consumers, and behavioural data from past users, we could identify the people who are most likely to make an online purchase after having been exposed to a banner ad.
When we talk about reinforcement learning, we’re going beyond simple predictions. Once we have an idea of what might happen in the future, then collect the data on what actually happened, the predictive model can be updated or enriched to become even better at predicting future events. So, it’s a dynamic model in a process of continual improvement, becoming ever more precise. The recommendation tools used by Amazon and Netflix are great examples of this. If Amazon gives you a purchase recommendation that doesn’t match your interests or situation, the tool will learn and adapt based on the behaviour it observes with the objective of giving you better recommendations the next time. Facebook’s facial recognition tool, the one that recognizes your friends’ faces in your photos, also uses ML algorithms. If the name the tool suggests doesn’t match the person in the photo, the algorithm stores that information to improve for next time. Today, the level of successful recognition has reached 98%, demonstrating the power of ML.
Machine learning requires advanced skills both in math and technology. It’s the kingdom of data scientists, defined by Josh Wills, Director of Data Engineering at Slack, as a “person who is better at statistics than any software engineer and better at software engineering than any statistician.” That’s why Adviso has integrated pure data scientists into its teams to apply this expertise to digital marketing, and more specifically to digital media campaigns.
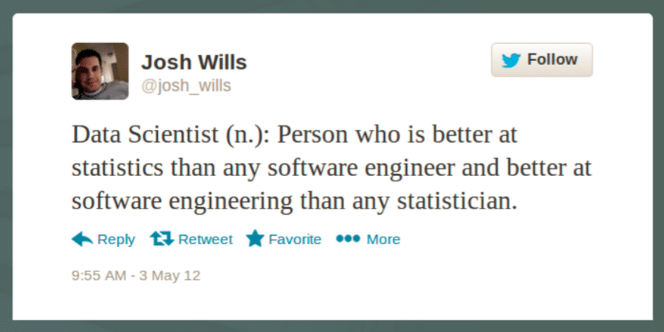
2 - How can using machine learning in digital marketing give advertisers a competitive advantage?
Machine learning is already used extensively in digital marketing – it’s at the heart of programmatic advertising. But today, ML is mainly just used on the supplier side (Google, Facebook, theTradeDesk, and all DSPs). Extremely widespread tools like Google AdWords are largely supported by ML. It’s impact, therefore, has already been huge, and we can easily conclude that the vast majority of advertisers are currently benefiting from ML to optimize their digital media campaigns, through their use of the major tech platforms. However, since everyone is already using the ML of existing proprietary platforms, no company has a real edge on the competition.
The determining factor, then, will be when advertisers start developing their own ML practices for digital marketing, and using them as decision-making tools. An advertiser that has developed its own ML practice would have a competitive advantage, gained by having a powerful and customized decision-making tool. They would no longer be dependant on ML algorithms developed by technology platforms and used by advertisers across the board, including the competition. What’s more, if advertisers used their own data in their ML algorithms in addition to ad platform and market data, the gain in efficiency would be significant.
A parallel example would be pre-made cookie dough that you can buy at the grocery store. Every advertiser can buy this dough to make their cookies. The final product would be similar to that of the other advertisers who bought the same pre-made dough. That’s the same as what happens when advertisers use the algorithms included in major ad platforms to make decisions. On the other hand, if an advertiser makes their own cookie dough internally, using a rigorous methodology, they can create a unique and customized asset, adapted to their needs and context, and that would allow them to stand out from the competition with a better quality product.
There are some major advertisers, Macy’s, for example, who are paving the way for ML in digital marketing and positioning themselves as pioneers. They are already using ML to make decisions about their marketing budgets, media mix, the creative they use, and the optimization of their digital media campaigns. The revolution is already underway: ML is no longer just in the hands of technology platforms, but those of advertisers, too.
3 - In concrete terms, what can ML do to improve the performance of company initiatives and the customer experience?
Segmentation, audience optimization, and predicting conversions on the basis of audience type
ML can help predict whether a given consumer will respond favourably to a promotion or not. We will therefore be able to segment the market based on degree of audience qualification and conversion potential.
Prediction of Customer Lifetime Value (CLV) and disengagement
The ability to predict CLV would help companies optimize their CPA over the long term. It would allow companies to focus on the acquisition of higher-value consumers, and scale back efforts to less profitable consumer segments.
Optimizing creative, targeting, and personalization
ML can help companies better target qualified users, and personalize their ad messaging. This means that it would be easier for advertisers to show the right ads to the right customers, thereby reducing acquisition costs, and improving the customer experience and company reputation.
Bid adjustment
This is currently the most-used method on the market in the world of digital media. It’s the optimization method offered by publishers. Some offerings are starting to crop up in the buyers market.
Conversion optimization
By using information gathered from analytics platforms, it’s possible to collect user behaviour data for a website or application and pair it with external audience data to improve conversion prediction criteria for different users.
4 - Media innovation and data science: How does Adviso use machine learning to optimize the performance of digital media campaigns?
Adviso is currently working on ML projects to support the day-to-day work of our digital media specialists, and provide them with more effective tools for optimizing media campaigns. We are strong believers in the potential of ML and its benefits for our clients. That’s why we decided to provide training on the subject for all our digital media specialists, to stay on the cutting edge of innovation in this ever-evolving market.
The integration of ML into Adviso’s digital media approach touches on the following areas, among others:
- Proactive research and the identification of web audiences with a strong potential for performance, without being limited to standard criteria for human selection. Our methodology is based on the logic of Multi Armed Bandit Optimisation;
- Proposals for the cessation, modification, or replacement of ad targeting based on current and past performance data, with the aim of improving performance predicted by algorithms;
- Predicting the evolution of a campaign’s performance indicators, on the basis of the amount of time invested;
- Recommendation on specific words to use in ads, to optimize campaign performance;
- The prediction of the impact of a traditional mass campaign on the results of various digital campaigns.
As stated by Pascal-Philippe Bergeron, our practice lead for Media Innovation and Data Science, “The job of a campaign manager will change from a specialist in digital buying methods, to a ML algorithm coach.”
5 - A few myths about machine learning
ML is going to replace humans
Clearly, machines are able to process astronomical quantities of data, and do it faster and more reliably than humans. Humans are simply not able to read, analyse, or manage thousands, sometimes millions of lines of data on our own. Machines have therefore become our assistants, digesting information and making suggestions that guide our decision-making. As a result, humans will always play an essential role in defining goals, identifying the data that needs to be processed, and drawing informed or sensitive qualitative conclusions, among other things. Judgement is exclusively a human quality, not easily transferred to a machine.
ML won’t replace humans. It will allow us to become more efficient, and reduce the risk of human error inherent in certain tasks, and to focus on the indispensible high-value activities that complement the work done by machines.
ML is exempt from biases
It’s true that ML limits a certain number of human biases. However, as mentioned below, ML requires human participation for the initial selection of data, algorithms, and platforms used. As Nicolas Scott, a data scientist at Adviso, reminds us, “the quality of the predictions stemming from the use of ML depends on the quality of the data – or, as they say, garbage in, garbage out.” The majority of human biases are eliminated, but not all.
ML requires a team of internal data scientists
There’s no point in surrounding yourself with an army of internal data scientists for your first go-arounds in the world of ML for digital marketing. This can be done externally. Adviso developed its service offering with the idea of allowing companies to benefit from the competitive advantages ML can bring, without the need for internal teams. Whether the ML is done internally or externally, it’s always important to use tools to capture and store the data that’s processed by the algorithms.
In summary, the ultimate goal of using ML in digital marketing is to create a more personalized customer experience throughout the customer journey, with the goal of stimulating engagement, conversion and retention, and optimizing the costs and resources invested, thanks to more efficient practices guided by machine learning. Companies that master ML will be able to develop a sustainable and significant competitive advantage with their target markets.